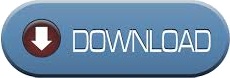
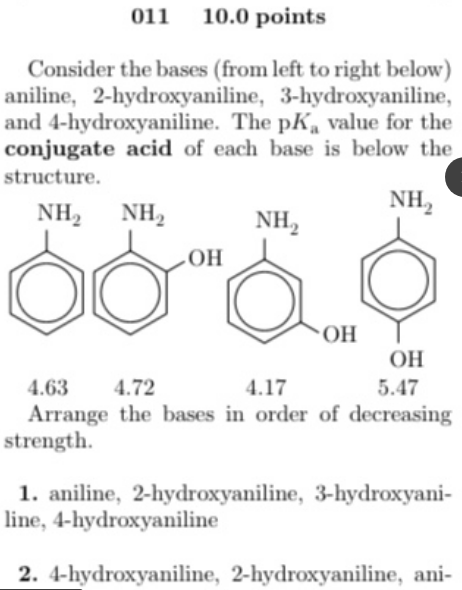
molecular mechanics energy, p K aetc.), and many different methods have been developed for their calculation. For this reason, the rules for determining partial atomic charges depend on their application (e.g. The partial atomic charges cannot be determined experimentally and they are also not quantum mechanical observables. A promising approach for p K aprediction is to use QSPR models which employ partial atomic charges as descriptors. However, p K avalues remain one of the most challenging physicochemical properties to predict.
#Pk value free#
Several approaches for p K aprediction have been developed, namely LFER (Linear Free Energy Relationships) methods, database methods, decision tree methods, ab initio quantum mechanical calculations, ANN (artificial neural networks) methods or QSPR (quantitative structure-property relationship) modelling. Therefore, both the research community and pharmaceutical companies are interested in the development of reliable and above all fast methods for p K aprediction. For these reasons, p K avalues are important for virtual screening. Moreover, the key physicochemical properties like lipophilicity, solubility, and permeability are all p K adependent.

The p K avalues have found application in many areas, such as the evaluation and optimization of candidate drug molecules, ADME profiling, pharmacokinetics, understanding of protein-ligand interactions, etc. The acid dissociation constant p K ais an important molecular property, and its values are of interest in pharmaceutical, chemical, biological and environmental research. In summary, EEM QSPR models constitute a fast and accurate p K aprediction approach that can be used in virtual screening.

The applicability of EEM QSPR models for other chemical classes was illustrated by a case study focused on carboxylic acids. The robustness of the EEM QSPR models was subsequently confirmed by cross-validation. Moreover, we found that the EEM QSPR models are not so strongly influenced by the selection of the charge calculation approach as the QM QSPR models. Furthermore, a big advantage of the EEM QSPR models is that their descriptors (i.e., EEM atomic charges) can be calculated markedly faster than the QM charge descriptors. As expected, QM QSPR models provided more accurate p K apredictions than the EEM QSPR models but the differences were not significant. We found that QSPR models employing the EEM charges proved as a good approach for the prediction of p K a(63% of these models had R 2 > 0.9, while the best had R 2 = 0.924). Afterwards, we calculated the quality criteria and evaluated all the QSPR models obtained. For each type of QM and EEM charges, we created one QSPR model employing charges from the non-dissociated molecules (three descriptor QSPR models), and one QSPR model based on charges from both dissociated and non-dissociated molecules (QSPR models with five descriptors). For all the molecules in the training set, we then calculated EEM charges using the 18 parameter sets, and the QM charges using the 8 above mentioned charge calculation schemes. Additionally, for each molecule we generated its dissociated form by removing the phenolic hydrogen.

Afterwards, we prepared a training set of 74 substituted phenols. Specifically, we collected 18 EEM parameter sets created for 8 different quantum mechanical (QM) charge calculation schemes. We have evaluated the p K aprediction capabilities of QSPR models based on empirical atomic charges calculated by the Electronegativity Equalization Method (EEM). The acid dissociation constant p K ais a very important molecular property, and there is a strong interest in the development of reliable and fast methods for p K aprediction.
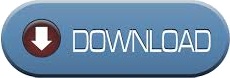